Key Applications of Computational Biomodeling
Computational biomodeling has numerous applications in biochemistry and related fields. Some of the key areas include:
Genetic Research
In genetic research, computational biomodeling helps scientists understand the effects of genetic mutations on protein structure and function. By modeling these mutations, researchers can identify potential causes of genetic disorders and develop targeted therapies. This approach is crucial for personalized medicine, where treatments are tailored to an individual’s genetic makeup.
Genetic Research in Computational Biomodeling
Genetic research in computational biomodeling combines the power of genetics and computational biology to understand complex biological systems. This interdisciplinary field uses computer simulations and models to predict the behavior of genetic networks and their impact on cellular functions. For more information, visit Genetic Research.
Applications of Computational Biomodeling
Computational biomodeling has a wide range of applications in genetic research:
- Disease Modeling: Simulating genetic mutations to study their impact on disease progression.
- Drug Development: Predicting the efficacy and safety of new drugs based on genetic interactions.
- Personalized Medicine: Tailoring treatments based on individual genetic profiles.
- Evolutionary Studies: Understanding genetic changes over time and their effects on populations.
Table: Key Tools in Computational Biomodeling
Tool | Description | Application |
---|---|---|
Bioinformatics Software | Software for analyzing biological data | Genomic sequencing, data analysis |
Mathematical Models | Equations representing biological processes | Predicting genetic interactions, modeling disease pathways |
Simulation Platforms | Virtual environments for testing hypotheses | Drug testing, virtual clinical trials |
Machine Learning Algorithms | Algorithms for pattern recognition | Genomic data analysis, predicting gene function |
Challenges in Genetic Research
Despite its potential, genetic research in computational biomodeling faces several challenges:
- Data Complexity: Managing and analyzing vast amounts of genetic data.
- Model Accuracy: Ensuring models accurately represent biological systems.
- Computational Power: Requiring significant computational resources for simulations.
- Ethical Considerations: Addressing privacy and ethical concerns in genetic research.
Future Directions
Future advancements in computational biomodeling and genetic research are likely to focus on:
- Integration of Multi-Omics Data: Combining genomics, proteomics, and metabolomics for comprehensive models.
- AI and Machine Learning: Leveraging artificial intelligence for predictive modeling and analysis.
- Personalized Medicine: Developing individualized treatment plans based on genetic information.
- Sustainable Practices: Using genetic insights for sustainable agriculture and environmental conservation.
Systems Biology
Systems biology is an interdisciplinary field that focuses on complex interactions within biological systems. Computational biomodeling is a crucial aspect of systems biology, employing mathematical and computational techniques to model and simulate biological processes. These models help scientists understand how individual components of a biological system interact to produce the behavior of the whole system. For more information, visit Systems Biology.
Applications of Computational Biomodeling
Computational biomodeling has numerous applications in research and medicine:
- Drug Discovery: Simulating biological pathways to identify potential drug targets and predict drug interactions. More details at NCBI.
- Personalized Medicine: Modeling patient-specific biological data to tailor treatments. Learn more at Nature.
- Understanding Diseases: Analyzing disease mechanisms through simulations to develop new therapeutic strategies. Visit ScienceDirect for further reading.
Key Components of Computational Biomodeling
The process of computational biomodeling involves several key components:
- Data Collection: Gathering quantitative data from experiments or databases.
- Model Construction: Developing mathematical models to represent biological systems.
- Simulation: Running simulations to predict the behavior of the system under various conditions.
- Validation: Comparing model predictions with experimental data to ensure accuracy.
- Analysis: Interpreting the results to draw meaningful biological conclusions.
Table: Key Computational Tools in Systems Biology
Tool | Description | Application |
---|---|---|
COPASI | Software for simulation and analysis of biochemical networks and their dynamics. | Biochemical network modeling |
CellML | Markup language for storing and sharing computer-based mathematical models. | Model sharing and reuse |
SBML | Standard format for representing computational models in systems biology. | Model representation |
SimBiology | MATLAB-based environment for modeling, simulating, and analyzing biological systems. | Modeling and simulation |
Challenges and Future Directions
Despite the advancements, computational biomodeling faces challenges such as data integration, model complexity, and computational power requirements. Future research aims to improve the accuracy of models and develop more user-friendly tools for researchers and clinicians. For the latest advancements, visit ScienceDirect.
Environmental Biotechnology
Environmental biotechnology leverages biological systems to address environmental challenges, such as pollution, waste management, and ecosystem restoration. Computational biomodeling plays a crucial role in this field by creating predictive models to understand and optimize biotechnological processes. For comprehensive details, visit Environmental Biotechnology.
Applications of Computational Biomodeling in Environmental Biotechnology
Computational biomodeling involves using mathematical and computational techniques to simulate biological processes. In environmental biotechnology, it helps in:
- Bioremediation: Modeling microbial communities to enhance the degradation of pollutants.
- Waste Treatment: Optimizing the microbial breakdown of organic waste in treatment plants.
- Bioenergy Production: Enhancing the efficiency of biofuel production through metabolic pathway analysis.
Key Techniques in Computational Biomodeling
Several computational techniques are essential in biomodeling for environmental biotechnology:
- Genome-scale Metabolic Modeling: Creating comprehensive models of metabolic networks to predict microbial behavior under different conditions.
- Agent-based Modeling: Simulating interactions between individual organisms within a microbial community to understand complex behaviors.
- Molecular Dynamics Simulations: Studying the physical movements of atoms and molecules to understand the structural dynamics of biomolecules.
Table: Computational Biomodeling Techniques in Environmental Biotechnology
Technique | Application | Benefits |
---|---|---|
Genome-scale Metabolic Modeling | Predicting microbial behavior | Optimizes bioprocesses, enhances pollutant degradation |
Agent-based Modeling | Simulating microbial community interactions | Improves understanding of community dynamics |
Molecular Dynamics Simulations | Studying biomolecule dynamics | Provides insights into structural behavior |
Case Studies and Success Stories
Numerous success stories highlight the effectiveness of computational biomodeling in environmental biotechnology:
- Oil Spill Bioremediation: Modeling microbial degradation of hydrocarbons to clean up oil spills, significantly reducing environmental impact.
- Wastewater Treatment Optimization: Using agent-based models to improve microbial efficiency in breaking down organic waste, enhancing treatment plant performance.
- Biofuel Production: Genome-scale modeling of algae and bacteria to increase lipid production for biodiesel, making biofuels more sustainable and cost-effective.
Challenges and Future Directions
While computational biomodeling offers tremendous potential, several challenges remain:
- Data Integration: Combining data from various sources and scales (genomics, proteomics, metabolomics) to create comprehensive models.
- Model Validation: Ensuring models accurately predict real-world behaviors, requiring extensive experimental validation.
- Computational Resources: High computational power is necessary to simulate complex biological systems accurately.
Future research aims to overcome these challenges by improving data integration techniques, enhancing model accuracy through advanced algorithms, and increasing computational efficiency. For more information, visit Future of Environmental Biotechnology.
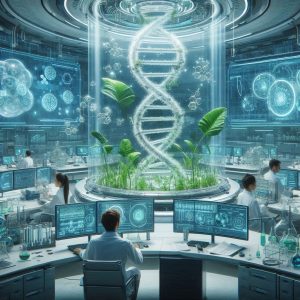
Environmental Biotechnology
Environmental biotechnology leverages biological systems to address environmental challenges, such as pollution, waste management, and ecosystem restoration. Computational biomodeling plays a crucial role in this field by creating predictive models to understand and optimize biotechnological processes. For comprehensive details, visit Environmental Biotechnology.
Applications of Computational Biomodeling in Environmental Biotechnology
Computational biomodeling involves using mathematical and computational techniques to simulate biological processes. In environmental biotechnology, it helps in:
- Bioremediation: Modeling microbial communities to enhance the degradation of pollutants.
- Waste Treatment: Optimizing the microbial breakdown of organic waste in treatment plants.
- Bioenergy Production: Enhancing the efficiency of biofuel production through metabolic pathway analysis.
Key Techniques in Computational Biomodeling
Several computational techniques are essential in biomodeling for environmental biotechnology:
- Genome-scale Metabolic Modeling: Creating comprehensive models of metabolic networks to predict microbial behavior under different conditions.
- Agent-based Modeling: Simulating interactions between individual organisms within a microbial community to understand complex behaviors.
- Molecular Dynamics Simulations: Studying the physical movements of atoms and molecules to understand the structural dynamics of biomolecules.
Table: Computational Biomodeling Techniques in Environmental Biotechnology
Technique | Application | Benefits |
---|---|---|
Genome-scale Metabolic Modeling | Predicting microbial behavior | Optimizes bioprocesses, enhances pollutant degradation |
Agent-based Modeling | Simulating microbial community interactions | Improves understanding of community dynamics |
Molecular Dynamics Simulations | Studying biomolecule dynamics | Provides insights into structural behavior |
Case Studies and Success Stories
Numerous success stories highlight the effectiveness of computational biomodeling in environmental biotechnology:
- Oil Spill Bioremediation: Modeling microbial degradation of hydrocarbons to clean up oil spills, significantly reducing environmental impact.
- Wastewater Treatment Optimization: Using agent-based models to improve microbial efficiency in breaking down organic waste, enhancing treatment plant performance.
- Biofuel Production: Genome-scale modeling of algae and bacteria to increase lipid production for biodiesel, making biofuels more sustainable and cost-effective.
Challenges and Future Directions
While computational biomodeling offers tremendous potential, several challenges remain:
- Data Integration: Combining data from various sources and scales (genomics, proteomics, metabolomics) to create comprehensive models.
- Model Validation: Ensuring models accurately predict real-world behaviors, requiring extensive experimental validation.
- Computational Resources: High computational power is necessary to simulate complex biological systems accurately.
Future research aims to overcome these challenges by improving data integration techniques, enhancing model accuracy through advanced algorithms, and increasing computational efficiency. For more information, visit Future of Environmental Biotechnology.
Neuroscience
In neuroscience, computational neural network models help understand brain function and the basis of neurological disorders. These models simulate the electrical and chemical processes within the brain, providing insights into how neural circuits operate and how they are affected by diseases such as Alzheimer’s and Parkinson’s.
For more on the applications of computational biomodeling, visit NCBI’s Overview of Applications.
Techniques in Computational Biomodeling
Several techniques are employed in computational biomodeling to simulate biological processes accurately. These include:
- Molecular Dynamics Simulations: This technique models the physical movements of atoms and molecules over time, providing insights into the structural dynamics of biomolecules.
- Monte Carlo Simulations: Used to predict the behavior of biomolecules by randomly sampling possible configurations and states.
- Quantitative Structure-Activity Relationship (QSAR): A method that correlates the chemical structure of molecules with their biological activity, aiding in drug design.
To explore more about these techniques, you can read Nature’s Review on Computational Techniques.
Challenges in Computational Biomodeling
Despite its potential, computational biomodeling faces several challenges, including:
- Complexity of Biological Systems: The vast number of variables and interactions in biological systems makes accurate modeling a significant challenge.
- Computational Power: High computational resources are required to simulate large and complex biological systems, often limiting the scope of studies.
- Data Accuracy: The accuracy of models depends on the quality of input data, which can sometimes be limited or imprecise.
For more insights into the challenges and future directions, refer to ScienceDirect’s Comprehensive Analysis.
Future Directions in Computational Biomodeling
The future of computational biomodeling is promising, with advancements in computational power, algorithms, and data availability driving the field forward. Emerging trends include:
- Integration with Artificial Intelligence: AI and machine learning are increasingly being used to enhance model accuracy and predict biological behaviors more efficiently.
- Personalized Medicine: Computational models are being tailored to individual genetic profiles, paving the way for personalized treatments and precision medicine.
- Real-time Simulations: Advances in computational speed are enabling real-time simulations of biological processes, which could revolutionize drug testing and development.
To stay updated on the latest advancements, check out Frontiers in Bioinformatics.
Applications of Computational Biomodeling
Application | Description | Resources |
---|---|---|
Drug Discovery | Predicting drug-target interactions to accelerate development and reduce costs | Nature Reviews |
Genetic Research | Understanding the effects of genetic mutations on protein function | Nature Reviews Genetics |
Systems Biology | Creating comprehensive models of biological systems | Nature Reviews Genetics |
Environmental Biotechnology | Optimizing microbial processes for bioremediation | ScienceDirect |
Agricultural Biotechnology | Developing genetically modified crops with improved traits | Frontiers in Plant Science |
Neuroscience | Modeling neural networks to understand brain function | Frontiers in Neuroscience |
For a detailed exploration of these applications, refer to Nature’s Review on Computational Biomodeling.
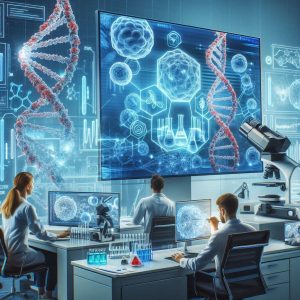
FAQs on Computational Biomodeling
What is Computational Biomodeling?
Computational biomodeling involves using mathematical and computational techniques to create models that simulate biological processes. These models help researchers understand complex systems and predict behaviors under various conditions. Learn more at Computational Biomodeling.
How is Computational Biomodeling Used in Environmental Biotechnology?
In environmental biotechnology, computational biomodeling is used to optimize bioprocesses such as bioremediation, waste treatment, and bioenergy production. It helps in predicting the outcomes of biotechnological interventions and improving their efficiency. For detailed applications, visit Environmental Biotechnology.
What are the Benefits of Computational Biomodeling?
Computational biomodeling provides several benefits, including enhanced understanding of biological systems, improved predictions of biotechnological processes, and the ability to test hypotheses in silico before conducting expensive and time-consuming experiments. Discover more at Nature.
What are the Challenges of Computational Biomodeling?
Some challenges include integrating data from diverse sources, validating models against experimental data, and requiring substantial computational resources to simulate complex systems accurately. Read about ongoing research at NCBI.
How Can I Learn More About Computational Biomodeling?
To learn more, you can explore academic journals, attend conferences, and participate in online courses focused on computational biology and bioinformatics. For resources, visit Coursera.
Disclaimer
The information provided in this article is for educational purposes only and is not intended as a substitute for professional advice or scientific research. The information provided in this article is for educational purposes only and is not intended as professional advice. While we strive to ensure the accuracy and relevance of the content, we cannot guarantee that all information is current or correct. Users should conduct their own research and consult with qualified professionals before making decisions based on the information provided.
Cautions
- Data Interpretation: Misinterpretation of computational models can lead to incorrect conclusions. Always validate models with experimental data.
- Computational Resources: High computational demands may limit the accessibility of advanced modeling techniques. Ensure adequate resources are available.
- Model Assumptions: Be aware of the assumptions and limitations inherent in any computational model. These can affect the accuracy and applicability of the results.
- Ethical Considerations: The use of computational modeling should be conducted ethically, with considerations for environmental impact and societal implications.